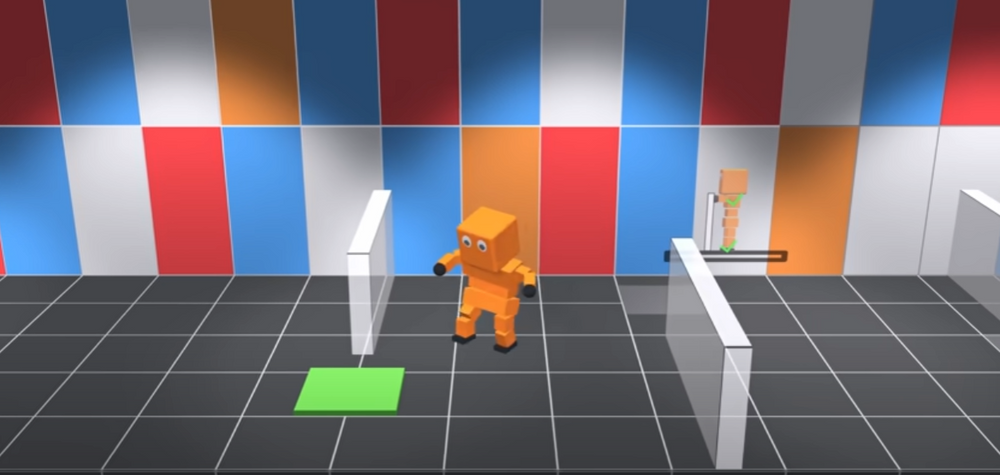
The Story of Albert and His Quest to Master Walking
In the world of artificial intelligence (AI), teaching a machine to walk may sound like a simple task. After all, we humans learn to walk effortlessly during our early childhood. However, when it comes to AI, it’s a whole different ball game. In this article, we delve into the fascinating journey of AI learning to walk through deep reinforcement learning, uncovering the challenges, breakthroughs, and real-life examples that illuminate the path to autonomous locomotion.
The Curious Case of Albert
Four months ago, a passionate AI enthusiast named Albert embarked on a daring mission. Frustrated by the limitations he observed in existing “AI learns to walk” videos, where robots either moved in strange, non-human ways or imitated human motion capture, Albert set out to do things differently. He aimed to train an AI to walk from scratch, without relying on external data, and in a truly human-like manner.
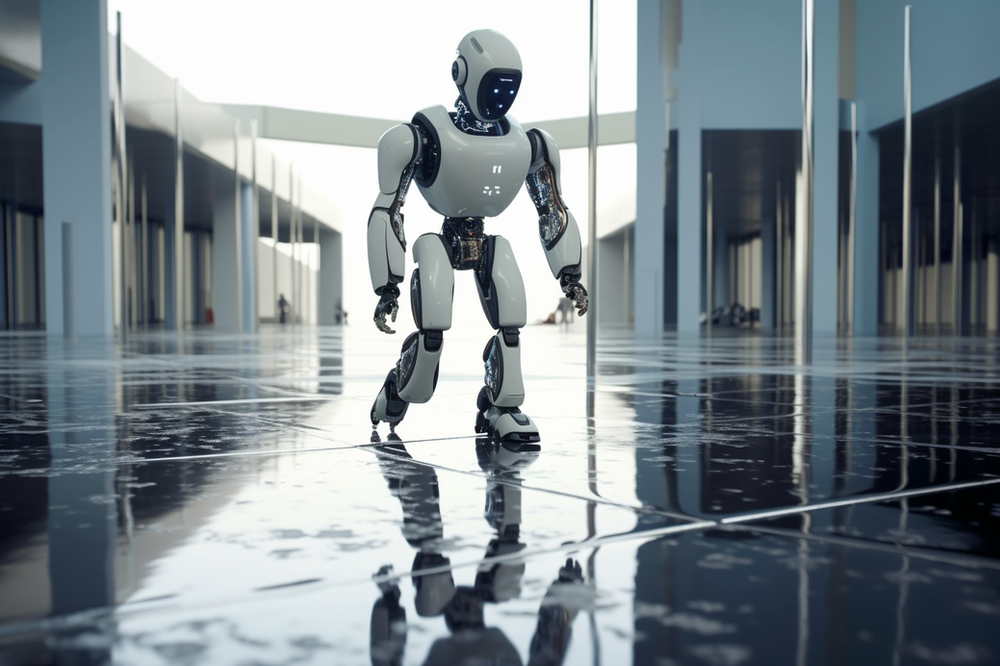
The Basics of Albert’s Journey
Albert’s adventure began with the creation of his artificial brain using Unity and ML-Agents. His neural network consisted of five layers, with the first layer receiving inputs such as limb positions, velocities, and contacts with the environment. The middle three layers, known as hidden layers, performed calculations to convert these inputs into actions. The final layer dictated the actions Albert should take. To train his brain, Albert employed the powerful reinforcement learning algorithm called proximal policy optimization (PPO).
A Peek Inside Albert’s Mind and Body
For each of Albert’s limbs, a multitude of information was fed into his brain. He received inputs about the limb’s position, velocity, angular velocity, contacts with the ground, walls, or obstacles, and the applied strength. Additionally, Albert was equipped with knowledge about the distance from each foot to the ground, the direction of the closest target, his body’s velocity, the distance between his chest and feet, and the time one foot spent in front of the other. With these inputs, Albert’s brain had the necessary information to make informed decisions about his actions.
Learning Through Rewards
Just like humans, Albert learned through a system of rewards and punishments. Each of his attempts was evaluated, and a score was calculated to determine the goodness of his actions. Increasing Albert’s score rewarded him, encouraging the behaviors that led to success, while decreasing his score punished him, discouraging unfavorable actions. Over time, Albert’s brain evolved and adapted, resembling the process of natural selection, where the best-performing Alberts were more likely to reproduce.
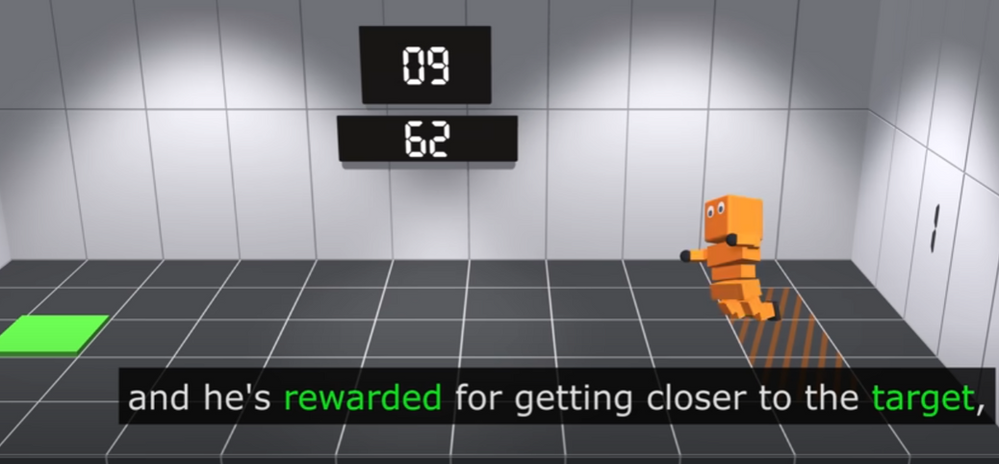
Unraveling the Reward Function
Albert’s training consisted of multiple rooms, each presenting new challenges and rewards. In Room 1, Albert was rewarded for moving towards the target and penalized for moving in the wrong direction. This led to a curious result: Albert discovered that performing the worm-like movement was the most efficient way to maximize his score. Room 2 introduced rewards for the contact of his limbs with the ground, focusing on his feet being in front of the other. This encouraged Albert to maintain balance and utilize his feet effectively.
In Room 3, Albert’s gait began to take shape, and he learned to turn. The height of his chest became a direct reward, motivating him to stand up as straight as possible.
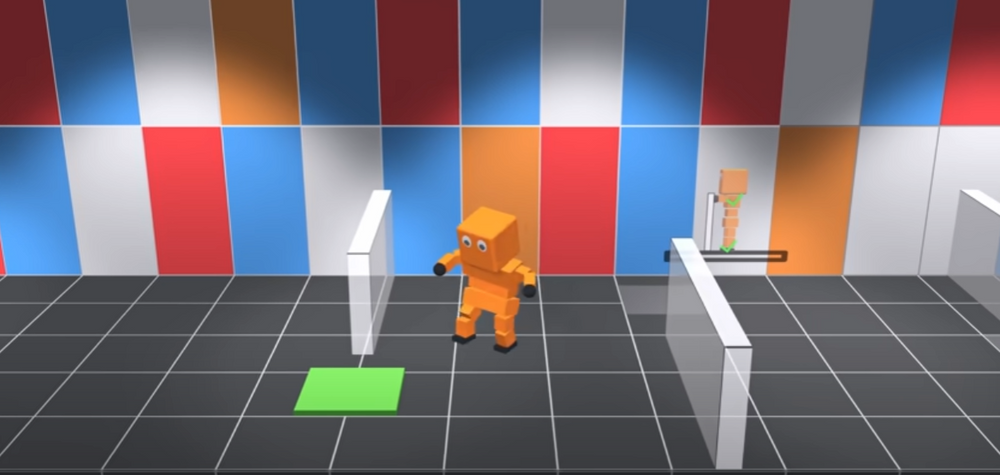
However, the challenge of using both feet proved to be the most difficult part of the project, requiring additional training in Room 4. Here, Albert took more steps, rewarded for maintaining a specific timer between his foot movements and encouraged to take larger steps.
The Triumph of Albert’s Strides
As Albert progressed through the rooms, his walking skills refined. Room 5 witnessed the culmination of Albert’s journey. In this final room, a minor adjustment to the reward function resulted in a significant improvement in his walking style. By rewarding the grounding of the front foot, Albert achieved a much smoother and more natural-looking walk. The video of his triumph showcased the culmination of months of training, perseverance, and continuous improvement.
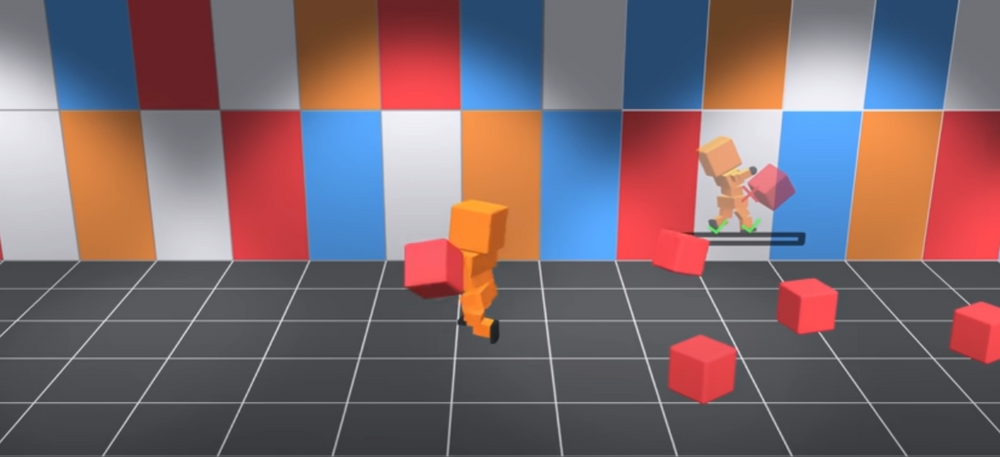
The Secrets of Albert’s Brain
Albert’s journey wasn’t without its challenges. One significant obstacle he faced was the issue of decaying plasticity. As his brain specialized in one room, training in the next room became difficult because he needed to unlearn the specialization from the previous environment. Resetting a random neuron periodically was the solution, helping Albert “forget” the specificities of the previous room without any noticeable impact. Although the process of resetting a single neuron within ML-Agents remains a challenge, Albert’s creator found a workaround by occasionally recording attempts trained from scratch and seamlessly integrating them into the training process.
The video above is from “AI Warehouse” and all rights belong to their respective owners. This amazing project was created by AI Warehouse, follow their channel for more videos like this.
From Tick Decisions to Smooth Motions
Albert’s training process evolved throughout the rooms. In Rooms 1 to 4, he was limited to making decisions every five game ticks, allowing him to commit to his actions for a longer duration. This constraint helped him develop a more careful and deliberate approach to movement. However, in the final room, this limitation was removed. By this stage, Albert had already learned to commit to his actions, and allowing him to make decisions every frame resulted in a smoother and more refined motion.
The Albert Army: Unleashing the Power of Multiplicity
Although you may see only one Albert in the video, there is a hidden army behind the scenes. Two hundred copies of Albert and the training room worked tirelessly in parallel to speed up the training process. This multiplication of Alberts allowed for a more efficient exploration of the solution space, leading to faster progress in acquiring the skill of walking.
Beyond Albert’s Adventure
While Albert’s story provides a captivating glimpse into the world of AI learning to walk, he is not alone in his quest. Real-life examples abound, demonstrating the power of deep reinforcement learning in robotics. One such example is Cassie, a pair of robot legs developed by Hybrid Robotics. Cassie’s journey involved training through reinforcement learning to acquire a range of movements, including walking in a crouch and carrying unexpected loads. The success of Cassie in autonomously walking across various terrains and recovering from external forces showcases the tremendous potential of deep reinforcement learning in real-world scenarios.
Walking Towards a Future of Possibilities
The journey of AI learning to walk through deep reinforcement learning is an exciting and transformative field. It not only holds the promise of enabling robots to navigate and interact with our world more effectively but also opens doors to a multitude of applications. From disaster response to industrial automation, the ability of robots to walk autonomously is a fundamental stepping stone toward a future where intelligent machines play an integral role in our lives.
As researchers continue to push the boundaries of AI and robotics, the quest for better, more agile walkers progresses. With each breakthrough and refinement of techniques, we edge closer to a reality where robots seamlessly integrate into our daily lives, providing assistance, performing complex tasks, and expanding the realm of what is possible.
In conclusion, Albert’s journey to master the art of walking through deep reinforcement learning exemplifies the incredible potential of AI in the field of robotics. From his humble beginnings to the triumph of his strides, Albert’s story showcases the power of perseverance, innovation, and continuous improvement. As we witness the progress in AI learning to walk, we find ourselves on the cusp of a revolution in robotics that will reshape industries, redefine possibilities, and ultimately transform the way we interact with intelligent machines.
Note: The views and opinions expressed by the author, or any people mentioned in this article, are for informational purposes only, and they do not constitute financial, investment, or other advice.
Relevant Articles:
New AI VideoGPT: Revolutionizing Video Generation with Transformers
Meet X.AI Elon Musk’s New AI Venture: The Future is Here
AI Clone Replaces Her For a Day: Joanna’s Bold ,Experiment